- Reduced out of stock inventory
- Improved customer satisfaction
- A more consistent balance of supply and demand
This article will explain how retailers can ensure these results by explaining AI's benefits within retail planning, the potential limitations to mitigate before implementation, and key takeaways regarding strategic adoption of AI within key retail planning processes.
How Are AI and Retail Connected?
Most retailers see the value of AI and are eager to implement it somewhere in some form, with the most common being customer service chatbots. For larger companies with complex supply chains, investing in AI solutions within Sales & Operations Planning (S&OP) is becoming more of a necessity than a novelty due to massive disruptions in supply chains and changing consumer demands.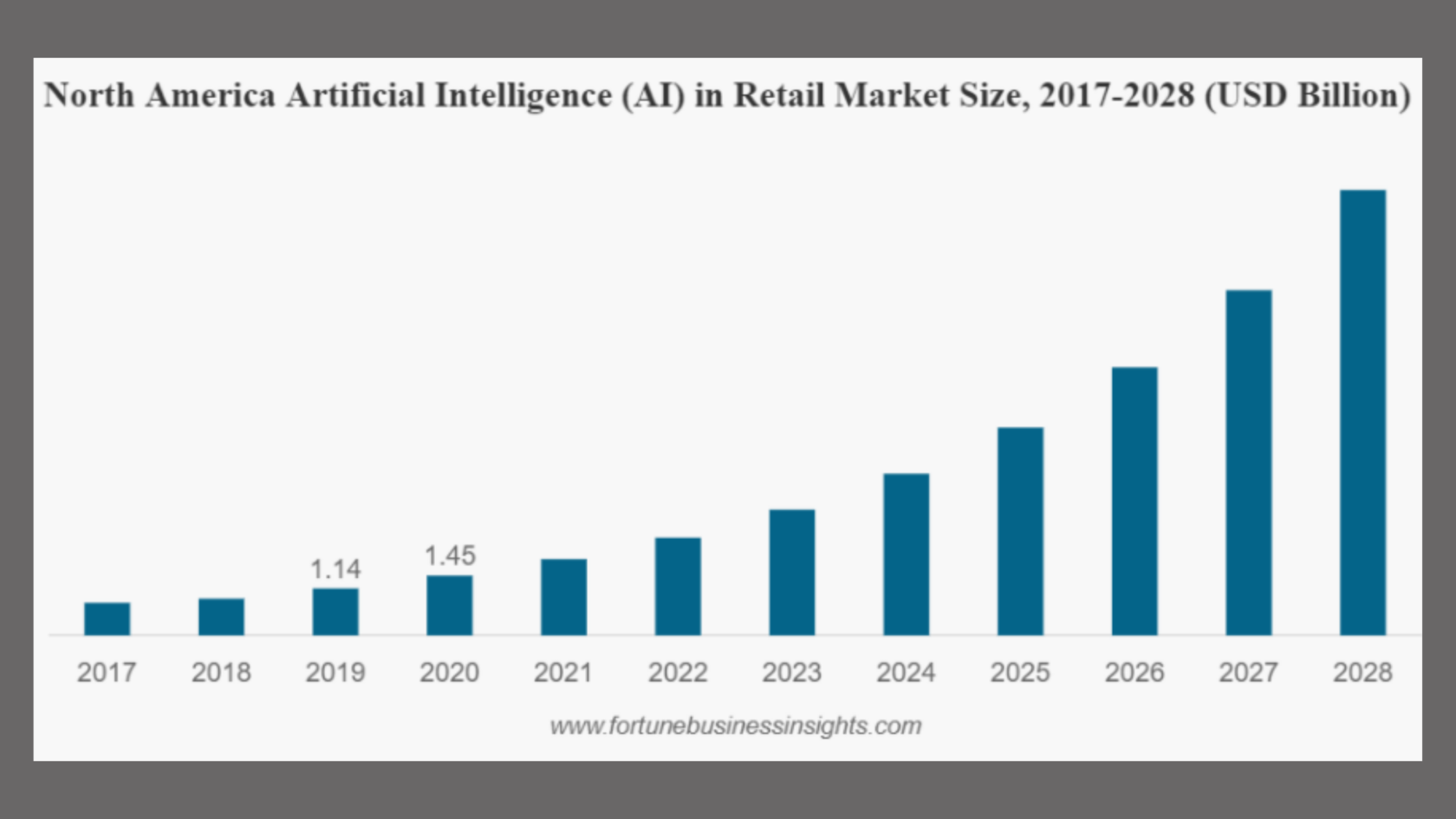
Retail planners across the world face the same two constant struggles: a) accurately predicting what a customer wants, and b) having enough inventory of those items to meet said customer demands.
In plain terms, these issues come down to supply chain management, with proper planning being a pillar to success. Retail planning refers to the steps retailers take to prepare their supply chain to meet key company goals. The retail planning process traditionally includes several tasks and sub-processes, with demand/sales forecasting and inventory management being two of the most promising areas that AI can provide tangible benefits.
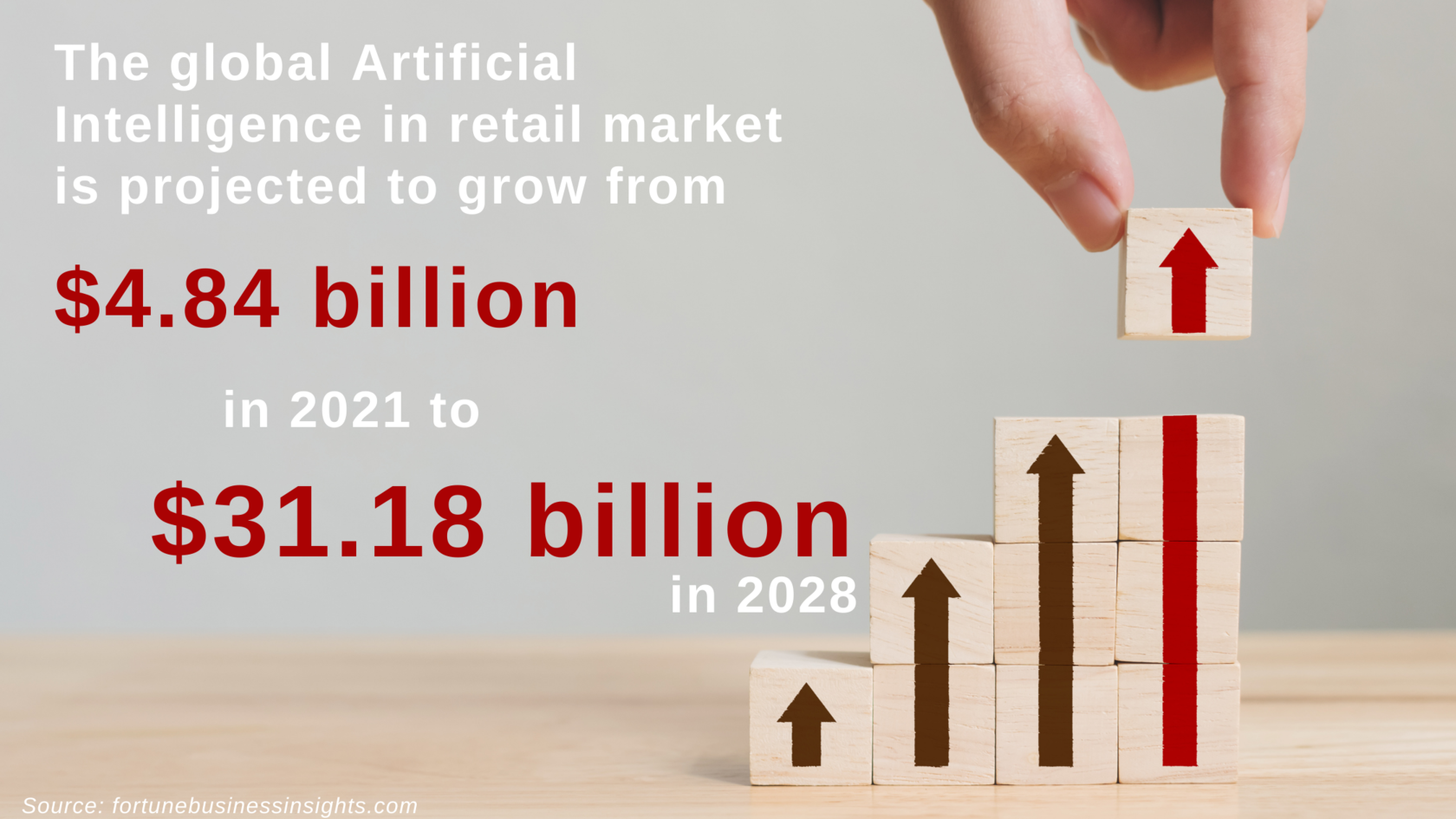
Forecasting Supply and Demand with AI
Everything affects retail demand, and demand affects everything else in retail planning. Demand and sales forecasts are at the forefront of preventing supply chain problems like out of stock and overstock inventory — if they’re inaccurate, all other forecasts and plans that follow will be as well.Despite its importance to supply chain management, the most common analytical tool for demand planning in retail is Excel sheets.
However, retail companies now have access to large data sets that can be used to train machine learning algorithms which in turn generate better forecasts for demand planning. Retail planners can use predictive analytics to identify patterns in customer data (e.g. purchase history, web browsing history), sales data, and big data from external sources (e.g. weather, social media).
This allows retail planners to identify patterns within demand and supply that humans alone cannot detect. For example, AI can make retail planners aware of the increased demand for certain products between noon and 1pm on weekdays, or can even predict how many customers are likely to purchase items in a specific department based on historical data. AI is also able to use retail data from various sources outside retail databases (i.e. big data) to contextualise historical data. This creates more accurate forecasts and allows retailers to visualise the customer journey.
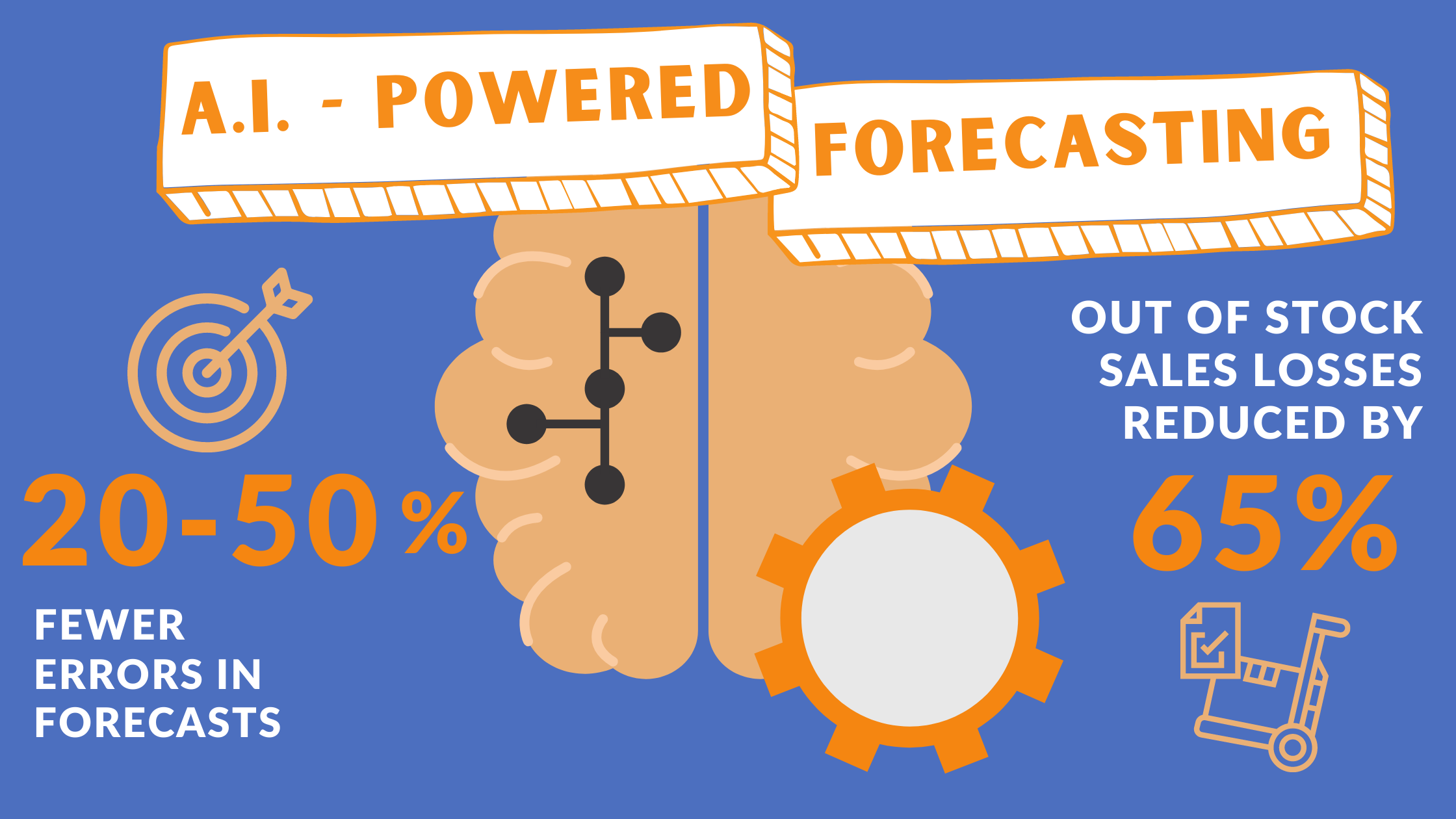
AI provides better, faster forecasts with less effort.
In most cases, multiple retail planners have to work endlessly to gather and analyse data in order to forecast supply and demand. Potential changes or possibilities regarding demand, objectives or external factors require them to repeat this process, and it's far from instantaneous. Using AI for forecasting models allows retail planners to dedicate their time to more pressing tasks. C-suites can make data-backed decisions faster considering a multitude of scenarios, providing the retail more flexibility within their supply chain strategy in case sudden disruption should occur.Retail needs Big Data most
Retail companies from grocers to high-end fashion brands need to anticipate and mitigate constant and often rapid changes in demand. Though historical data is integral to accurate forecasting, being able to consider changes that may not be reflected in past records is important in order to meet present customer expectations. Big data analysis gives retailers the ability to ensure their historical data is being analysed to create a more accurate visualisation of the future.A well-trained machine learning algorithm can identify the relationship between variables and dependencies, creating a model that retail planners can use to generate accurate retail forecasts and harmonise supply and demand. McKinsey reports that inventory reduction of 20-50% is possible with AI-powered demand forecasting, with lost sales due to out of stock inventory being reduced by up to 65%. Forecasting and modelling are also not limited to supply and demand — out of stock modelling, assortment planning, and many more aspects of retail planning can be augmented using AI.
Inventory Management in Retail Supply Chains with AI
AI's usefulness in retail planning doesn't stop at forecasting; retailers have the option to implement AI throughout the supply chain to provide better inventory management and data accuracy. A lack of supply chain visibility can prevent forecasts and analyses from being accurate due to poor quality data — for example, products not accounted for properly within warehouses when broken, lost, or stolen can result in faulty inventory data inputs. Using AI, retailers can access the plethora of data their warehouses produce to ensure they maintain a constant pulse on the movement of inventory and their planning teams have access to high-quality data.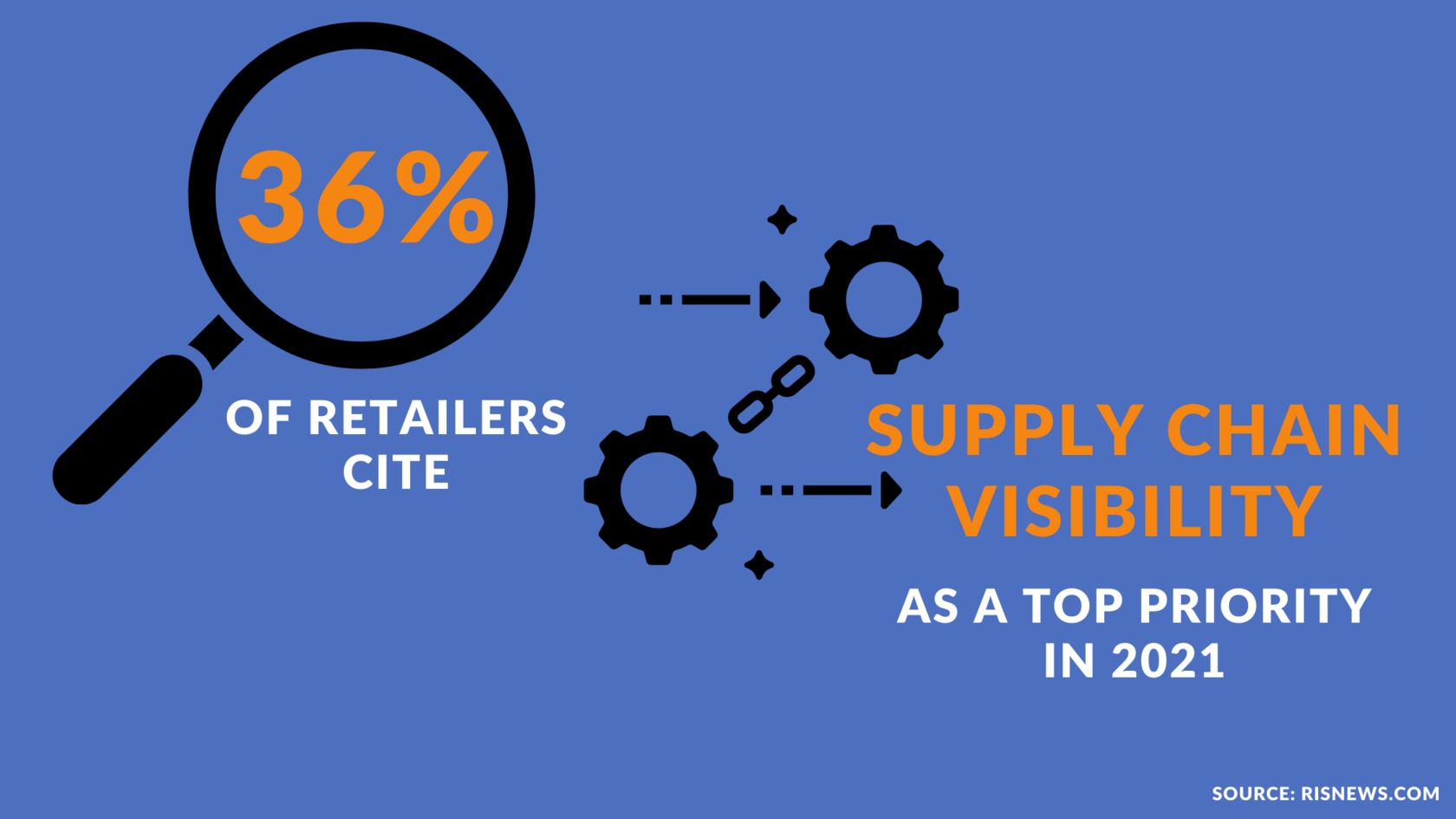
AI in warehouses
Amazon isn't the only retailer that can leverage the power of AI within its warehouses. In fact, AI-powered solutions for retail warehouse management are becoming increasingly affordable and accessible to mid-size and even small retail businesses. When combined with IoT and robotics, warehouse management can be completely automated to reduce errors and lesson injuries to staff from repetitive movements and physically demanding tasks.For retailers looking towards less extensive investments, scanners can use AI to automatically detect barcodes via mobile devices and send this real-time data straight to the ERP system even if the staff has limited internet access. Management and c-level executives maintain instant access to this information at their fingertips, making inventory management more transparent.
In-store
Having fast, accurate data collection on the front lines of retail also has notable benefits. AI-powered bots scanning shop aisles can provide retail planning teams with real-time insights on what products are most in demand. This information can be used to adjust forecasts for optimal product availability and increase sales conversion. Though the pillar-like bots still make customers scratch their heads as they scoot around stores, one retail chain found that within a matter of months, it had detected 14 times as many out-of-stock products, and could report shelved quantities that takes a human worker over one full workday in less than a few hours.Online
ECommerce is no stranger to AI, but its applications for digital storefronts are constantly expanding. AI-powered chatbots are one of the most popular retail AI applications for good reason. They can provide an individualised customer experience while automating simple tasks such as product recommendations, order tracking, and customer service inquiries.Digital storefronts can also use machine learning algorithms to understand how customers interact with their websites and personalise their online shopping experiences. All of this information can be collected and used for retail planning processes like demand forecasting, assortments, and marketing.
A.I.-Powered Retail Technologies: Limitations & Considerations
The race towards digital transformation often shifts the conversation around AI away from the fact that it is a tool, not an outcome. A company that adds AI to its toolkit is not automatically better off than it was before because the results AI provides are only as good as its inputs. This creates three areas of consideration for companies before implementing AI for retail planning: data, objectives, and people.Data: quantity, freshness, accuracy, and governance of data
Objectives: Specificity and size of AI inputs in relation to company objectives
People: The technological literacy of team members required to leverage AI effectively to avoid errors, bias, and/or underperformance
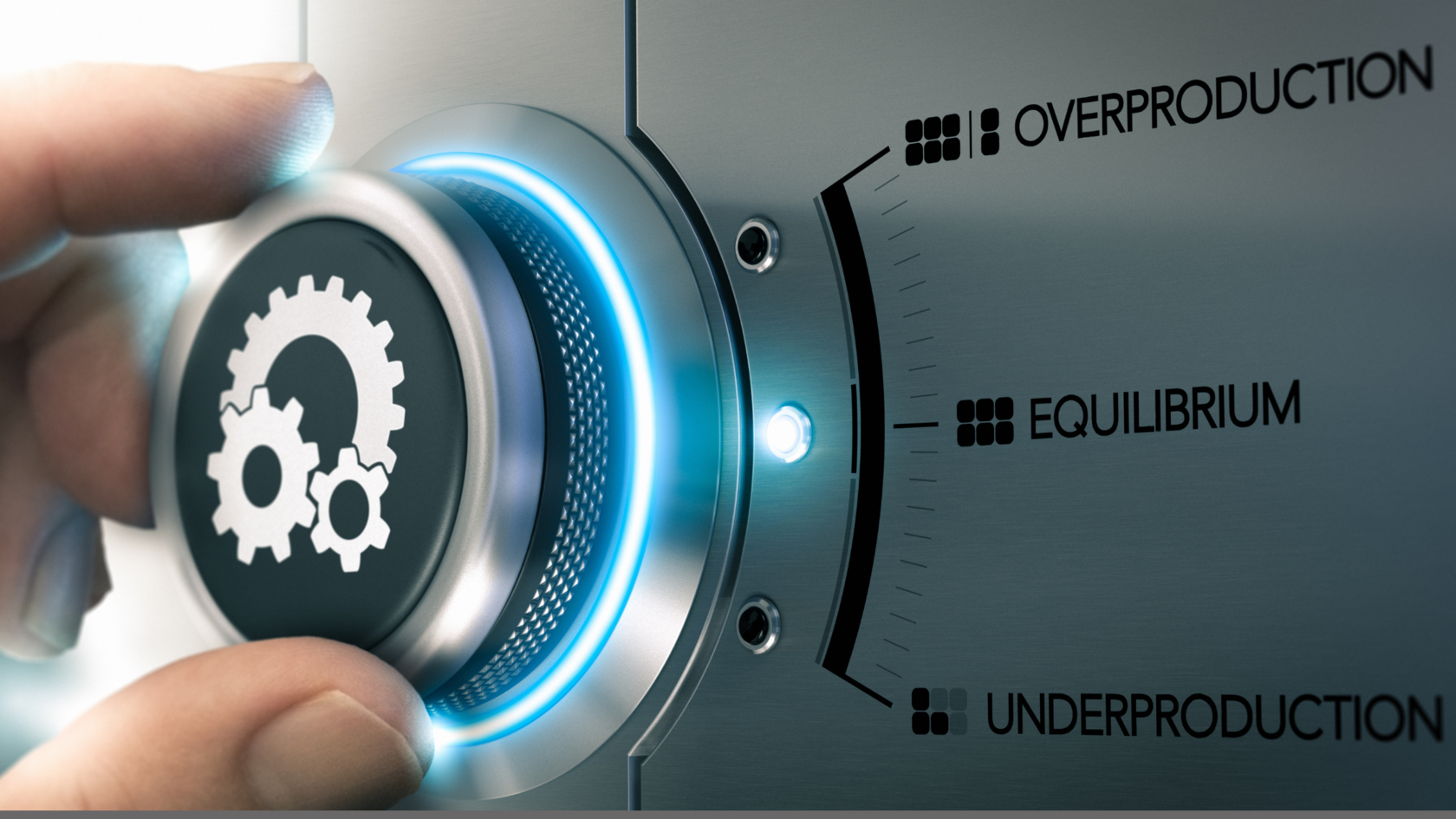
Data
The results AI can achieve are heavily dependent on the quality and quantity of data it is given. Having data scientists on hand with specialised experience in AI software provides massive long-term payoffs, as they will be able to determine the best inputs for specific goals, ensure data is constantly updated to maintain accuracy, and can help avoid errors within datasets. In many cases, AI software providers can provide this expertise on a retailer's behalf in the form of application-managed services. Hiring consultants specialised in AI application managed services is also an option for retailers with limited qualified personnel.Proper data formatting, storage, and governance are vital for AI to be able to perform tasks properly. In the case of social data used to analyse customer behaviour or consumer trends, proper governance is integral to avoiding bias. Bias can arise due to "information bubbles" like specific social platforms, which can cause an unequal representation of specific demographics due to its user base. Bias can also be caused by a lack of diversity within IT teams or system users unintentionally creating bias when determining what data to include or exclude.
Objectives
Oftentimes AI outputs are viewed as answers to how a company can achieve its goals, but it's much more accurate to consider AI as a method of testing hypotheses to determine the best course of action. Knowing this, larger objectives need to be deconstructed and mapped out into smaller, more specific definitions in order to leverage AI's capabilities. Learning to define proper objectives for AI in retail planning is imperative especially at the start, as this will determine how AI "understands" the information it's given and it sets the foundation for how it can define larger goals as it matures.A simplified example of objective mapping in retail planning is using AI to forecast demand. The retailer's larger goals may be to increase revenue, reduce the likelihood of items going out of stock, or reduce waste from unsold perishables. The defined objectives for the AI, however, would be to predict the number of units sold for a specific product on a specific day, in different seasons/weather conditions, and so on.
Employees
A team's technical sophistication and AI literacy should be considered before implementing AI-powered software to ensure key players in the planning process have the necessary skill sets to leverage it effectively. Oftentimes, this involves extensive training or in the case of larger companies, hiring new team members with the experience required. Alternatively, the help of expert consultancies can alleviate the often steep learning curve or potential setbacks that often occurs during training and implementation.Without this skillset or training, managers and executives may not use AI outputs to the fullest extent because they're not versed in how the software produced it. Alternatively, retail planners and data managers can make mistakes within data inputs and objective-setting that produce unpredictable or inaccurate results.
As mentioned previously, the team itself should also be diverse enough to produce results without significant bias.According to VentureBeat, a Columbia University study found that "the more homogenous the [engineering] team is, the more likely it is that a given prediction error will appear." This seemingly small detail could end up negatively impacting the accuracy and reliability of AI outputs across several areas of planning, from customer behaviour to demand forecasting.
Integrating AI into Retail Planning Processes: Key Takeaways
AI will undoubtedly play a key role in retailing of the future — in many verticals, it already does. Before taking the leap into AI-powered retail planning technology, consider the larger questions behind its intended purpose in your company:What can AI help you achieve?
It's unrealistic to expect fantastic results with AI without a clear idea of exactly what your company is aiming to achieve. Similar to how AI needs specific objective mapping, break down lofty or vague goals into smaller, more specific objectives that AI can assist with.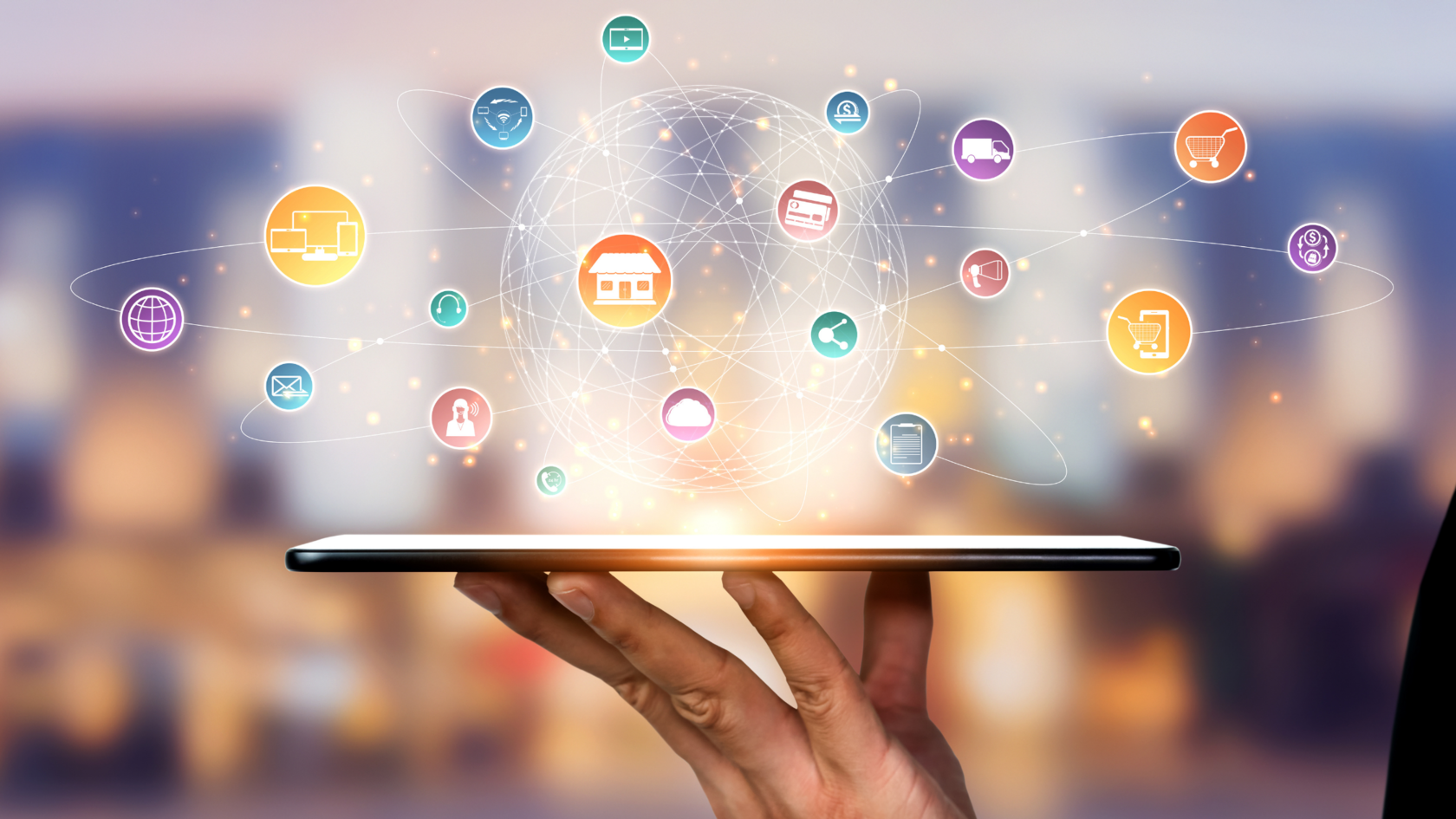
Does your company have what AI needs to do its job?
It's important to assess where retail planning technology fits into your company's larger structure. This can be in a literal sense regarding integration within existing ERP systems, for example. Developing a proof of concept also requires ensuring that your company's data management team can acquire the data you would need for your desired AI implementation and that all stakeholders from top to bottom have a sufficient understanding of how it works.
How and what are you going to choose?
As with any retail planning technology, AI will only deliver the best results when done properly. Before implementing AI into your retail planning processes, research various solutions and how they work to ensure yours is the right fit for your objectives. It's also important to choose an AI provider that can provide strong support, whether it be regular consultation or extensive training. Consider expert consultation services to get unbiased, experienced input on the best fit for your operations and how to compare solutions or providers.Bringing AI into the retail planning process can seem daunting, but with careful consideration of your company's needs, it can be an incredibly valuable asset. By taking the time to set specific, achievable objectives and ensuring a team is in place with the requisite skill set, any business can successfully integrate AI into retail planning and improve its operations.